—
Insights
The Hidden Carbon Footprint of AI: Understanding and Addressing the Environmental Impact of Artificial Intelligence
19 December 2024
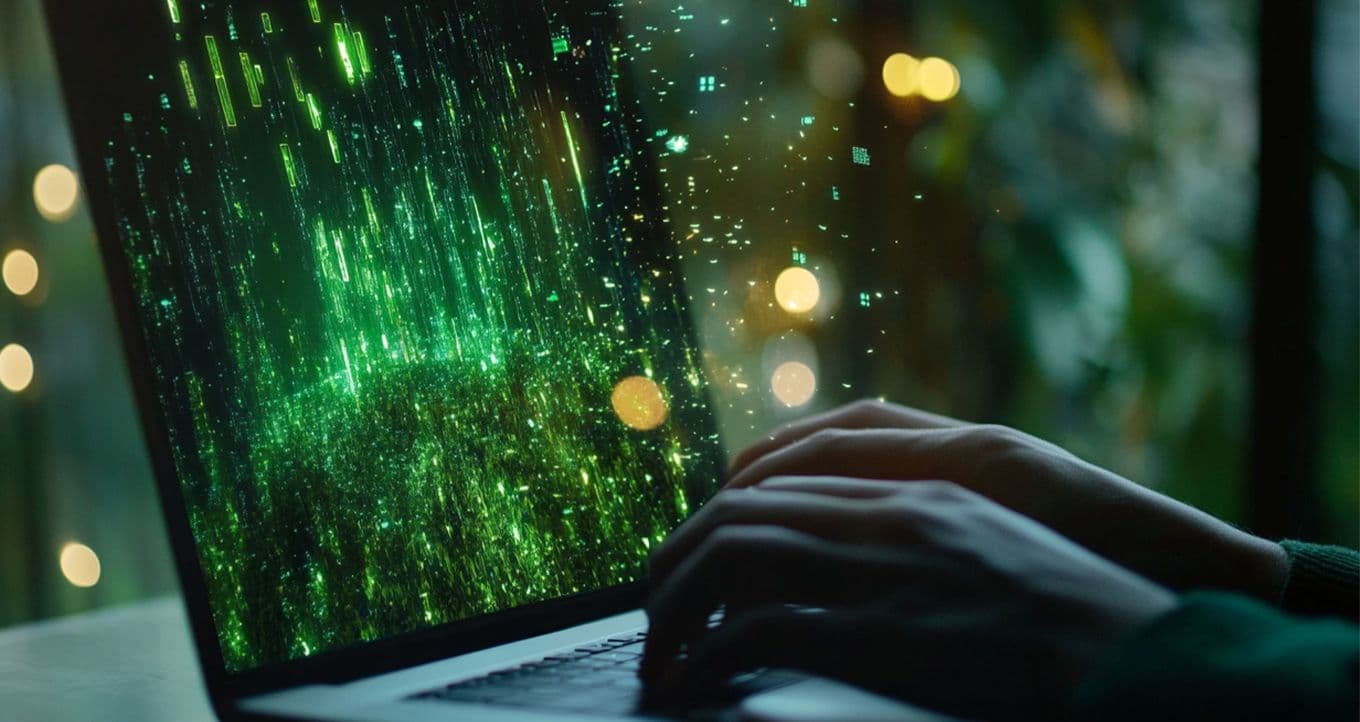
As artificial intelligence continues to revolutionise industries worldwide, a critical but often overlooked aspect is emerging: its environmental impact. This comprehensive analysis reveals the true carbon footprint of AI systems, from their energy-intensive training processes to daily operations.
The Environmental Cost of Training AI Models
The development of large AI models represents one of the most significant contributors to the technology's carbon footprint. The numbers are striking:
GPT-3, one of the most well-known language models, produced approximately 552 metric tons of CO2 equivalent (CO2e) during its training phase alone. To put this into perspective, this equals the annual emissions of 120 gasoline-powered cars.
A typical large language model training process consumes around 626,155 kWh of energy, equivalent to the annual electricity usage of 57 average American homes. The environmental impact becomes even more significant when examining the neural architecture search phase, which can emit up to 284 tons of CO2e. Let's break down the numbers for a standard AI training process:
- Power consumption for a 100 GPU cluster: 100 kW
- Average training duration: 7 days
- Total energy consumption: 16,800 kWh
- Resulting carbon emissions: 7.56 metric tons CO2e
Daily Operations: The Continuous Carbon Cost
While training represents the initial environmental impact, daily operations (inference) create an ongoing carbon footprint:
Single Query Impact:
- One GPT-3 query: 0.2-0.9g CO2e
- Daily emissions for 1 million queries: 200-900 kg CO2e
- Annual inference emissions for a popular AI service: 73-325 metric tons CO2e
The Data Center Factor
Behind every AI system lies a complex network of data centres, which contribute significantly to the overall environmental impact:
Global AI Data Center Statistics:
- Total annual power consumption: 200-250 TWh (2-3% of global electricity)
- Energy distribution:
- Servers and storage: 45% (90-112.5 TWh)
- Cooling systems: 40% (80-100 TWh)
- Power distribution: 15% (30-37.5 TWh)
- Average Power Usage Effectiveness (PUE): 1.57
- Carbon intensity: 0.4-0.7 kg CO2e per kWh (varies by location)
Solutions for a Sustainable AI Future
The AI industry is actively developing solutions to reduce its environmental impact. Here's how different approaches can cut emissions:
Carbon-Aware Computing Implementation of smart scheduling can reduce carbon intensity by 30-45%. For a large AI training run, this means:
- Original emissions: 7.56 metric tons CO2e
- After carbon-aware scheduling: 4.54 metric tons CO2e
- Total reduction: 3.02 metric tons CO2e
Efficient Model Design optimised architecture and training approaches can decrease computing power needs by 20-80%:
- Original power consumption: 16,800 kWh
- After optimisation: 3,360-13,440 kWh
- Potential carbon reduction: 1.51-6.05 metric tons CO2e
Green Data Centres switching to renewable energy can reduce operational carbon emissions by up to 100%:
- Traditional data centre: 0.7 kg CO2e per kWh
- Green data centre: 0-0.1 kg CO2e per kWh
- Potential annual reduction per data centre: 35,040 metric tons CO2e
The Combined Impact of Sustainable Practices
When implementing all these solutions together, the results are significant:
Starting point: 7.56 metric tons CO2e per training run After optimisations:
- Carbon-aware scheduling: -40% (4.54 metric tons CO2e)
- Efficient architecture: -50% (2.27 metric tons CO2e)
- Renewable energy: -90% (0.23 metric tons CO2e) Total reduction: 96.9%
Future Outlook and Industry Responsibility
As AI technology continues to evolve, the industry must prioritise environmental sustainability. Companies and organisations should:
- Implement comprehensive emissions monitoring
- Invest in energy-efficient infrastructure
- Develop standardised sustainability metrics
- Partner with renewable energy providers
- Support research into more efficient AI architectures
Conclusion
While AI's carbon footprint presents significant environmental challenges, the industry's growing commitment to sustainability offers promise. Through continued innovation and implementation of green technologies, we can work toward an AI future that balances technological advancement with environmental responsibility.
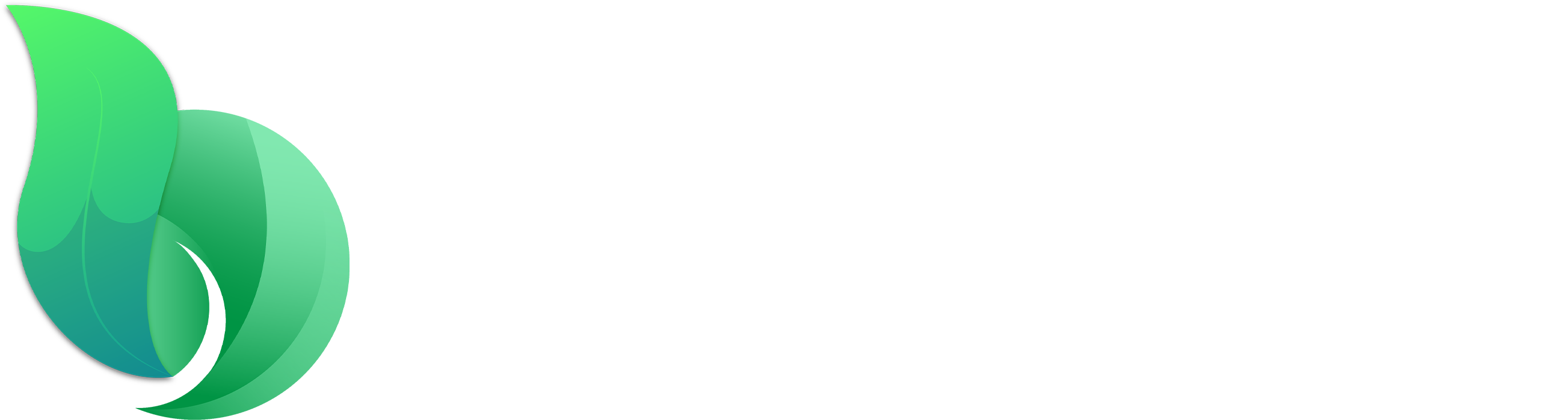
Do you know your website's carbon emissions?
We will never share your personal information with third parties.
170,000+ website pages analysed
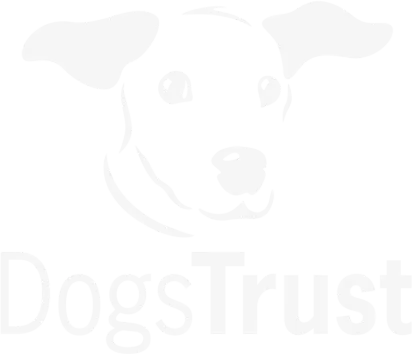
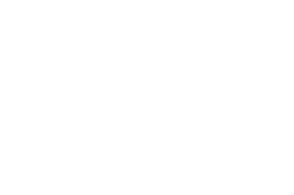
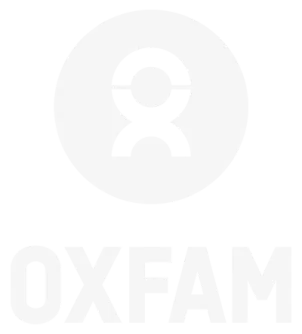
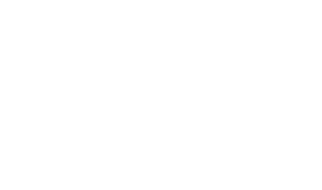
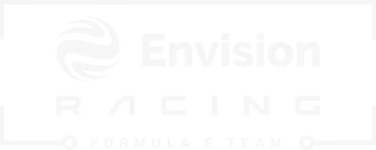